Models
The Encord API allows you to interact with the Encord model features. Our model library includes state-of-the-art classification, object detection, segmentation, and pose estimation models.
Creating a model row
The easiest way to get started with creating a model row is to navigate to the 'models' tab in your project. Create a model and set parameters accordingly.
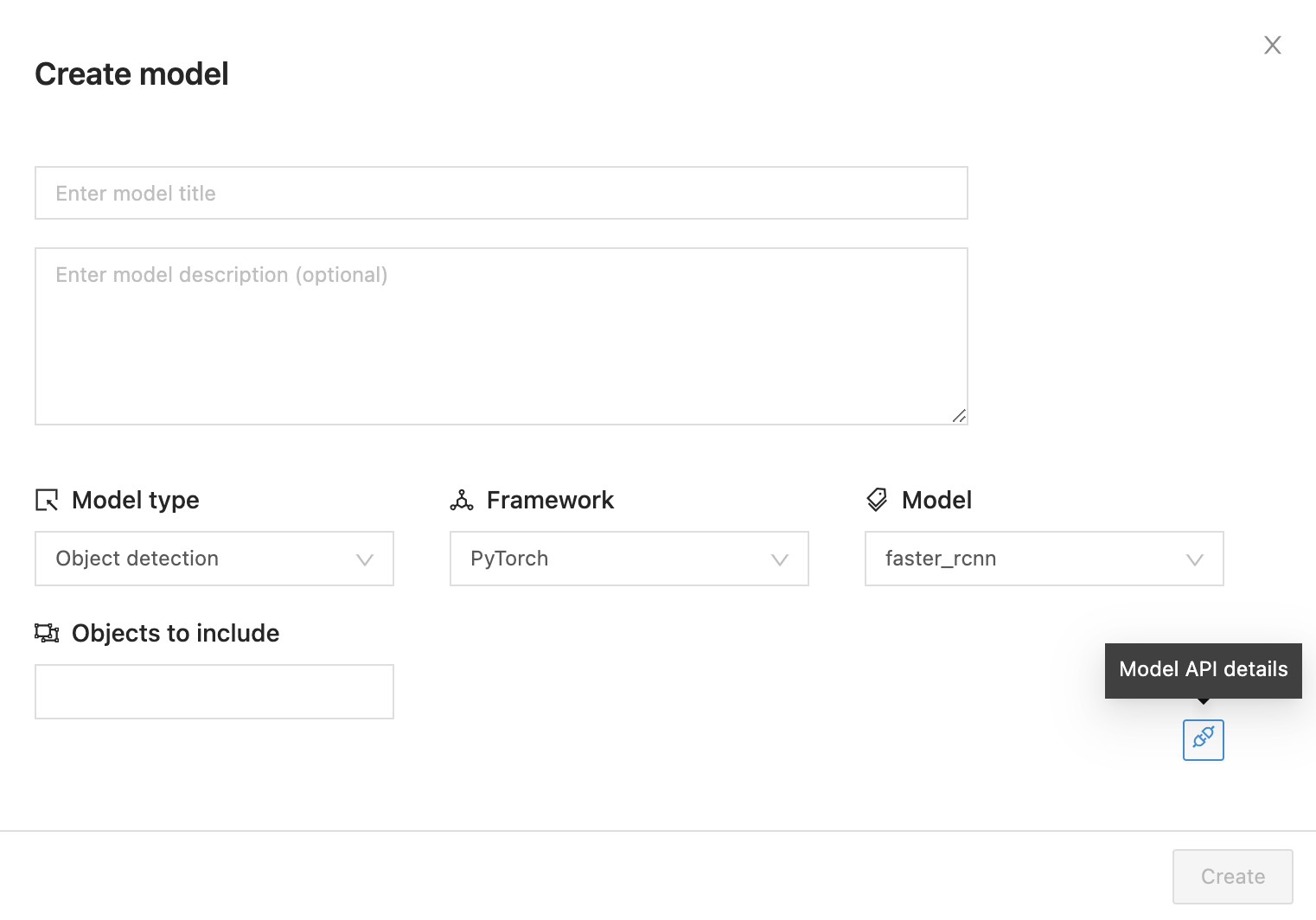
Click on the 'Model API details' button to toggle a code snippet with create model row API details when you are happy with your selected parameters. The feature ID's (featureHash
uid's) can also be fetched from the project editor information.
- Node
- curl
var axios = require('axios');
var data = JSON.stringify(
{
"query_type":"model",
"query_method": "PUT",
"values": {
"uid": null,
"payload": {
"model_operation": 2,
"model_parameters": {
"title": "sample_title",
"description": "sample_description",
"features":["feature_uid_1", "feature_uid_2"],
"model": "sample_model"
}
}
}
}
);
var config = {
method: 'post',
url: 'https://api.encord.com/public',
headers: {
'Content-Type': 'application/json',
'ResourceID': '<project_id>',
'Authorization': '<project_api_key>',
'Accept': 'application/json'
},
data : data
};
axios(config)
.then(function (response) {
console.log(JSON.stringify(response.data));
})
.catch(function (error) {
console.log(error);
});
curl --location --request POST 'https://api.encord.com/public' \
--header 'Content-Type: application/json' \
--header 'ResourceID: <project_id>' \
--header 'Authorization: <project_api_key>' \
--header 'Accept: application/json' \
--data-raw '{
"query_type": "model",
"query_method": "PUT",
"values": {
"uid": null,
"payload": {
"model_operation": 2,
"model_parameters": {
"title": "sample_title",
"description": "sample_description",
"features": [
"feature_uid_1",
"feature_uid_2"
],
"model": "sample_model"
}
}
}
}'
The request returns a model ID (model_hash
uid).
The following models are available, and have to be passed as strings.
"resnet18"
"resnet34"
"resnet50"
"resnet101"
"resnet152"
"vgg16"
"vgg19"
"yolov5"
"faster_rcnn"
"mask_rcnn"
Training
Navigate to the 'models' tab in your project to get started with model training. Start by creating a model row using the Encord API or by following the guidelines here. You can also use an existing model by clicking on the 'train' button.
Navigate through the training flow and set parameters accordingly.
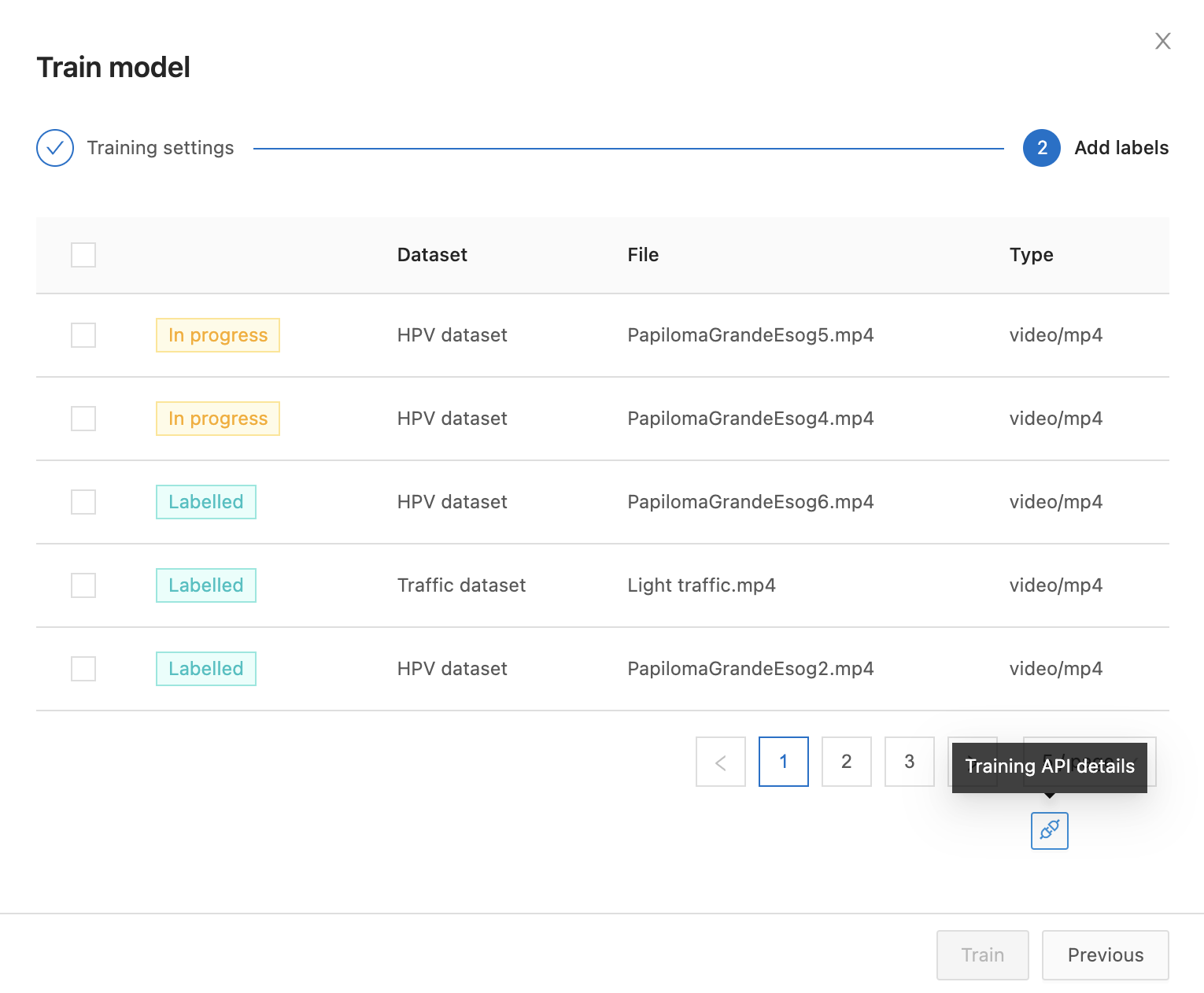
Click on the 'Training API details' button to toggle a code snippet with model training API details when you are happy with your selected label rows and parameters. The label row ID's (label_hash
uid's) can also be fetched from the project information.
- Node
- curl
var axios = require('axios');
var data = JSON.stringify(
{
"query_type": "model",
"query_method": "POST",
"values": {
"uid":"<model_hash>",
"payload": {
"model_operation": 1,
"model_parameters": {
"label_rows": [
"label_row_uid_1",
"label_row_uid_2"
],
"epochs": num_epochs,
"batch_size": batch_size,
"weights": {
"model": "sample_model",
"training_config_link": "",
"training_weights_link": ""
},
"device":"cuda"
}
}
}
}
);
var config = {
method: 'post',
url: 'https://api.encord.com/public',
headers: {
'Content-Type': 'application/json',
'ResourceID': '<project_id>',
'Authorization': '<project_api_key>',
'Accept': 'application/json'
},
data : data
};
axios(config)
.then(function (response) {
console.log(JSON.stringify(response.data));
})
.catch(function (error) {
console.log(error);
});
curl --location --request POST 'https://api.encord.com/public' \
--header 'Content-Type: application/json' \
--header 'ResourceID: <project_id>' \
--header 'Authorization: <project_api_key>' \
--header 'Accept: application/json' \
--data-raw '{
"query_type": "model",
"query_method": "POST",
"values": {
"uid": "<model_hash>",
"payload": {
"model_operation": 1,
"model_parameters": {
"label_rows": [
"label_row_uid_1",
"label_row_uid_2"
],
"epochs": num_epochs,
"batch_size": batch_size,
"weights": {
"model": "sample_model",
"training_config_link": "sample_config_link",
"training_weights_link": "sample_weights_link"
},
"device": "cuda"
}
}
}
}'
It is important that the weights used for the model training is compatible with the created model. For example, if you have created a faster_rcnn
object detection model, you should use faster_rcnn
weights.
The following pre-trained weights are available for training.
var fast_ai = {
"model": "fast_ai",
"training_config_link": "",
"training_weights_link": ""
}
var yolov5x = {
"model": "yolov5",
"training_config_link": "https://cord-models.s3.eu-west-2.amazonaws.com/Yolo/yolov5x.yaml",
"training_weights_link": "https://cord-models.s3.eu-west-2.amazonaws.com/Yolo/yolov5x.pt"
}
var yolov5s = {
"model": "yolov5",
"training_config_link": "https://cord-models.s3.eu-west-2.amazonaws.com/Yolo/yolov5s.yaml",
"training_weights_link": "https://cord-models.s3.eu-west-2.amazonaws.com/Yolo/yolov5s.pt"
}
var faster_rcnn_R_50_C4_1x = {
"model": "faster_rcnn",
"training_config_link": "https://cord-models.s3.eu-west-2.amazonaws.com/Detectron/config/faster_rcnn_R_50_C4_1x.yaml",
"training_weights_link": "https://cord-models.s3.eu-west-2.amazonaws.com/Detectron/weights/model_final_721ade.pkl"
}
var faster_rcnn_R_50_DC5_1x = {
"model": "faster_rcnn",
"training_config_link": "https://cord-models.s3.eu-west-2.amazonaws.com/Detectron/config/faster_rcnn_R_50_DC5_1x.yaml",
"training_weights_link": "https://cord-models.s3.eu-west-2.amazonaws.com/Detectron/weights/model_final_51d356.pkl"
}
var faster_rcnn_R_50_FPN_1x = {
"model": "faster_rcnn",
"training_config_link": "https://cord-models.s3.eu-west-2.amazonaws.com/Detectron/config/faster_rcnn_R_50_FPN_1x.yaml",
"training_weights_link": "https://cord-models.s3.eu-west-2.amazonaws.com/Detectron/weights/model_final_b275ba.pkl"
}
var faster_rcnn_R_50_C4_3x = {
"model": "faster_rcnn",
"training_config_link": "https://cord-models.s3.eu-west-2.amazonaws.com/Detectron/config/faster_rcnn_R_50_C4_3x.yaml",
"training_weights_link": "https://cord-models.s3.eu-west-2.amazonaws.com/Detectron/weights/model_final_f97cb7.pkl"
}
var faster_rcnn_R_50_DC5_3x = {
"model": "faster_rcnn",
"training_config_link": "https://cord-models.s3.eu-west-2.amazonaws.com/Detectron/config/faster_rcnn_R_50_DC5_3x.yaml",
"training_weights_link": "https://cord-models.s3.eu-west-2.amazonaws.com/Detectron/weights/model_final_68d202.pkl"
}
var faster_rcnn_R_50_FPN_3x = {
"model": "faster_rcnn",
"training_config_link": "https://cord-models.s3.eu-west-2.amazonaws.com/Detectron/config/faster_rcnn_R_50_FPN_3x.yaml",
"training_weights_link": "https://cord-models.s3.eu-west-2.amazonaws.com/Detectron/weights/model_final_280758.pkl"
}
var faster_rcnn_R_101_C4_3x = {
"model": "faster_rcnn",
"training_config_link": "https://cord-models.s3.eu-west-2.amazonaws.com/Detectron/config/faster_rcnn_R_101_C4_3x.yaml",
"training_weights_link": "https://cord-models.s3.eu-west-2.amazonaws.com/Detectron/weights/model_final_298dad.pkl"
}
var faster_rcnn_R_101_DC5_3x = {
"model": "faster_rcnn",
"training_config_link": "https://cord-models.s3.eu-west-2.amazonaws.com/Detectron/config/faster_rcnn_R_101_DC5_3x.yaml",
"training_weights_link": "https://cord-models.s3.eu-west-2.amazonaws.com/Detectron/weights/model_final_3e0943.pkl"
}
var faster_rcnn_R_101_FPN_3x = {
"model": "faster_rcnn",
"training_config_link": "https://cord-models.s3.eu-west-2.amazonaws.com/Detectron/config/faster_rcnn_R_101_FPN_3x.yaml",
"training_weights_link": "https://cord-models.s3.eu-west-2.amazonaws.com/Detectron/weights/model_final_f6e8b1.pkl"
}
var faster_rcnn_X_101_32x8d_FPN_3x = {
"model": "faster_rcnn",
"training_config_link": "https://cord-models.s3.eu-west-2.amazonaws.com/Detectron/config/faster_rcnn_X_101_32x8d_FPN_3x.yaml",
"training_weights_link": "https://cord-models.s3.eu-west-2.amazonaws.com/Detectron/weights/model_final_68b088.pkl"
}
var mask_rcnn_X_101_32x8d_FPN_3x = {
"model": "mask_rcnn",
"training_config_link": "https://cord-models.s3.eu-west-2.amazonaws.com/Detectron/config/mask_rcnn_X_101_32x8d_FPN_3x.yaml",
"training_weights_link": "https://cord-models.s3.eu-west-2.amazonaws.com/Detectron/weights/model_final_2d9806.pkl"
}
var mask_rcnn_R_50_C4_1x = {
"model": "mask_rcnn",
"training_config_link": "https://cord-models.s3.eu-west-2.amazonaws.com/Detectron/config/mask_rcnn_R_50_C4_1x.yaml",
"training_weights_link": "https://cord-models.s3.eu-west-2.amazonaws.com/Detectron/weights/model_final_9243eb.pkl"
}
var mask_rcnn_R_50_C4_3x = {
"model": "mask_rcnn",
"training_config_link": "https://cord-models.s3.eu-west-2.amazonaws.com/Detectron/config/mask_rcnn_R_50_C4_3x.yaml",
"training_weights_link": "https://cord-models.s3.eu-west-2.amazonaws.com/Detectron/weights/model_final_4ce675.pkl"
}
var mask_rcnn_R_101_FPN_3x = {
"model": "mask_rcnn",
"training_config_link": "https://cord-models.s3.eu-west-2.amazonaws.com/Detectron/config/mask_rcnn_R_101_FPN_3x.yaml",
"training_weights_link": "https://cord-models.s3.eu-west-2.amazonaws.com/Detectron/weights/model_final_a3ec72.pkl"
}
Inference
To get started with model inference, make sure you have created a project API key with model.inference
added to access scopes. The easiest way to get started with model inference is to navigate to the 'models' tab in your project.
Open the model training log for the model you would like to use for inference.
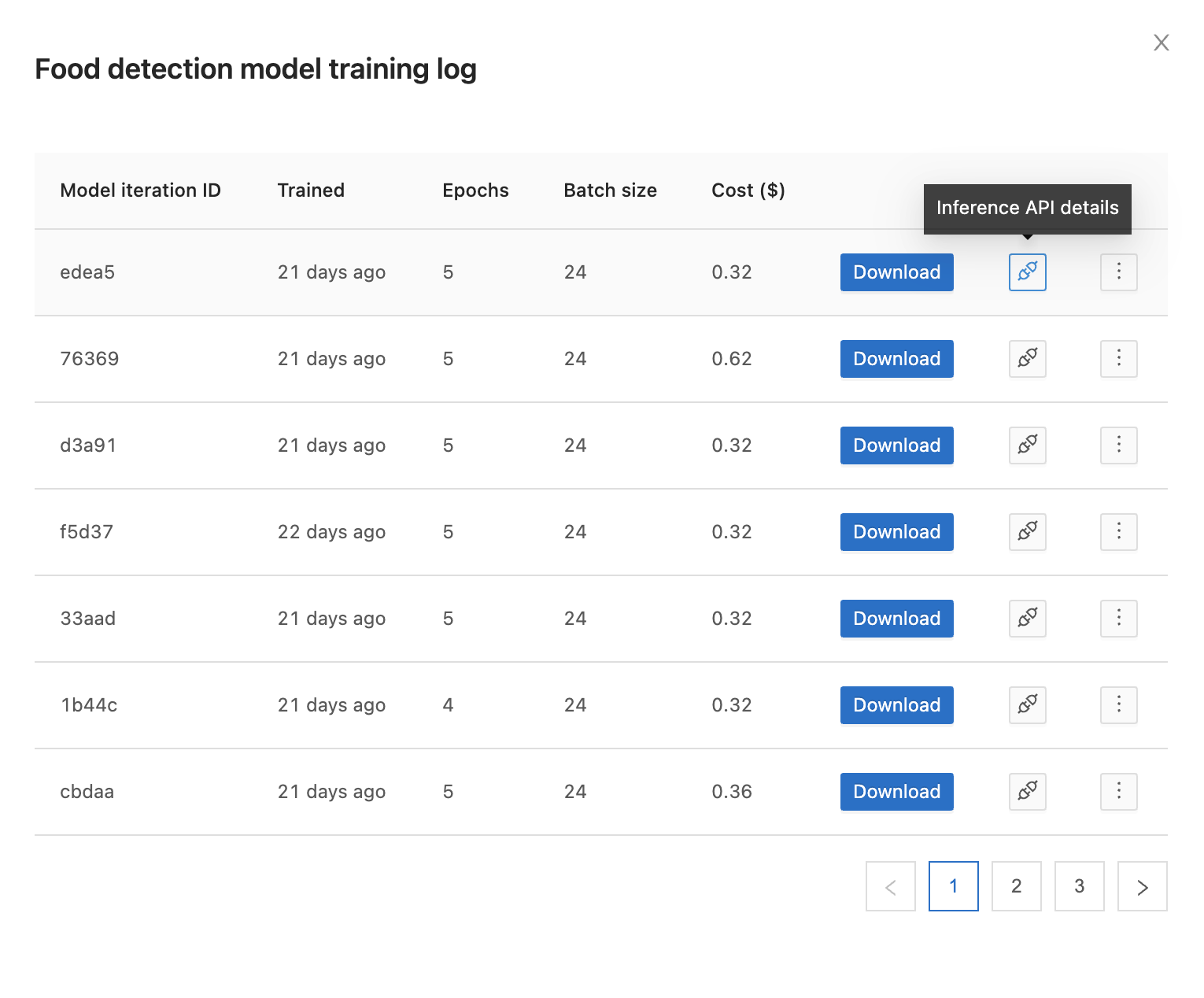
Click the 'inference API details' icon next to the download button to toggle a code snippet with model inference details. The model inference API accepts a list of base64 encoded strings with an uid.
You can define confidence and intersection-over-union (IoU) thresholds. The default confidence threshold is set to 0.6
, and the default IoU threshold is set to 0.3
.
For video inference, you must define a detection frame range.
Object UID allocation (tracking) is disabled by default and is defined by the parameter allocation_enabled
.
- Node
- curl
Equivalently using NodeJS with Axios and with a single example file, using the Node fs
package to parse the file into a base64 string:
var axios = require('axios')
var fs = require('fs');
var conf_thresh = 0.6;
var iou_thresh = 0.3;
function modelInference (filePath, modelIterationId) {
var file = fs.readFileSync(filePath[0], {encoding: 'base64'});
var data = {
"query_type": 'model',
"query_method": "POST",
"values": {
"uid": modelIterationId,
"payload": {
"model_operation": 0,
"model_parameters": {
"files": [
{
"uid": Math.random(),
"base64_str": file,
}
],
"conf_thresh": conf_thresh,
"iou_thresh": iou_thresh,
"device": "cuda",
"detection_frame_range": [],
"allocation_enabled": false
}
}
}
}
var config = {
method: 'post',
url: 'https://api.encord.com/public',
headers: {
'Content-Type': 'application/json',
'ResourceID': '<project_id>',
'Authorization': '<project_api_key>',
'Accept': 'application/json'
},
data : data
};
axios(config)
.then(function (response) {
console.log(JSON.stringify(response.data));
})
.catch(function (error) {
console.log(error);
});
}
modelInference(['path/to/file/1.jpg'], '<model_iteration_id>')
curl --location --request POST 'https://api.encord.com/public' \
--header 'Content-Type: application/json' \
--header 'ResourceID: <project_id>' \
--header 'Authorization: <project_api_key>' \
--header 'Accept: application/json' \
--data-raw '{
"query_type": "model",
"query_method": "POST",
"values": {
"uid": "<model_iteration_id>",
"payload": {
"model_operation": 0,
"model_parameters": {
"files": [
{
"uid": file_identifier,
"base64_str": base64_str_1,
},
{
"uid": file_identifier,
"base64_str": base64_str_2,
}
],
"conf_thresh": conf_thresh,
"iou_thresh": iou_thresh,
"device": "cuda",
"detection_frame_range": [],
"allocation_enabled": false
}
}
}
}'